Carolyn E. Schwartz, ScD1,2
1DeltaQuest Foundation, Inc., Concord, MA, USA
2Departments of Medicine and Orthopaedic Surgery, Tufts University Medical School, Boston, MA, USA
*Paraphrased from “A journey of a thousand miles begins with a single step,” which is attributed to Chinese philosopher Lao Tzu.
As a post-doc at Harvard Medical School in the early 1990’s, I attended the regular Tuesday luncheon meetings with all of the healthcare providers treating multiple sclerosis (MS) patients. In search of eligible patients for my clinical trial testing the effectiveness of a coping-skills group intervention I had developed to teach coping flexibility , I would listen as each provider went through the list of patients they had seen in the weekly MS clinic that morning, hoping that one or more would be deemed someone I could invite to participate in my two-year trial (1). Over time, and as I got to know the faces and the stories behind the names being discussed in the clinic, I saw a discrepancy in how the patients were described by their healthcare provider versus how they saw themselves. For example, sometimes they were described as better off than they themselves expressed to me. Other times, the opposite. I realized that MS outcome measures were missing important information about what mattered to patients. This led to my developing several measures that expanded the notion of MS disability and symptoms, and of factors related to patients’ resilience over time. These measures have since been translated into many languages and used in many studies, including clinical trials, registry studies, and observational studies.
Building on these comprehensive measures, I started to think about how to integrate these disparate perspectives into a method for analyzing clinical trials data. It seemed to me that considering only one perspective (i.e., the so-called “objective” perspective of the clinician) missed important information. So, I developed a multi-attribute utility approach called the Extended Quality Adjusted Time Without Symptoms and Toxicity (Q-TWiST) method , which extended the Q-TWiST methodology used in cancer clinical trials to a chronic disease context (2). The Extended Q-TWiST integrated the perspectives of the patient, the provider and social cost into a single number (a utility). It used quality-of-life scores reflecting condition-specific patient-reported indicators of physical, emotional, social, and cognitive functioning, as well as an indicator of social cost (e.g., time lost from work), and a clinical assessment (e.g., an index of neurological systems functioning). Patient-reported importance ratings for each domain were used as weights at each time point, and the end result provided an estimate of how much good quality time the experimental treatment yielded as compared to the comparison treatment (i.e., in Quality-Adjusted-Life-Years or QALYs). While the method was successfully applied in MS and epilepsy, I stumbled upon a realization that gave me pause: What was important depended on what was not doing well, not necessarily reflecting a consistent hierarchy of domains for each patient over time.
This thought led me to response shift.
At my first (and the first) ISOQOL meeting, I heard a talk on response shift that left me trembling with excitement. Although the speaker treated response shift as measurement error that needed to be adjusted out of analysis, I realized that these response-shift effects were themselves meaningful and important information that needed to be captured by reliable and valid measures, and perhaps even taught (i.e., as a psychosocial intervention). I realized that results from clinical trials were likely hidden by response-shift effects: If patients in one arm had the more effective treatment, they might shift in what was important to them over time. Conversely, if patients in the less effective treatment arm did not fare well over the course of the clinical trial, they might activate different coping approaches to maintain a homeostasis (stability) in their day-to-day experience. In both cases, the treatment effects could become less detectable. And my hunch was that response-shift effects were more likely hiding the benefits of treatment, not leading to exaggerated estimates of such.
To get from these epiphanies to the recent findings from our ISOQOL 2022 plenary presentation, “Listening to the elephant in the room: Response-Shift effects in clinical trials research”(3) took 30 years of persistent effort. There were many necessary steps before we could be in a position to test the hunch-driven hypothesis. We had to develop response-shift theory (4), and methods that could be used in secondary analysis (i.e., solely statistical ) and in new data collection. We had to evaluate existing methods to determine whether they were worth their salt (spoiler alert: they weren’t), and to create new or extend existing methods to estimate response shift effects . I found myself on a path focused on measuring how people think about quality of life (“cognitive-appraisal processes”), studying these processes in over 6000 medically ill patients with a range of conditions, coding qualitative data on thousands of patients, and eventually determining that adequately measuring cognitive-appraisal processes entailed a different class of measurement than usual psychometric or clinimetric tools (5). Eventually, this long and winding path led to several secondary analyses of clinical trials data where we documented that when response shift effects were considered, the detected treatment effects became larger and, in some cases, particularly for mental-health outcomes.
As co-chair of ISOQOL’s Response Shift Special Interest Group (SIG), I led a small group of international collaborators to do a scoping review of the clinical trials literature to ask whether response-shift effects mattered in clinical trials outcomes (3). From an initial set of 2148 unique references, we examined 25 clinical trials, of which 17 were retained after applying exclusion criteria. Ten of these 17 were adequately powered, of which seven revealed clinically important response-shift effects that made the intervention look stronger. These findings supported the importance of considering response-shift effects in clinical trials and using the right methods – ones that are reliable, valid, and tap what is relevant to the construct – with an adequate sample size for the selected method (i.e., adequate statistical power).
So now that this hunch of 30+ years ago has been “proven” right, am I done?
No! It is only pointing to the next steps on this path. There are many trials that have yet to consider response-shift effects. Getting trialists not only to consider response shift but also to measure what is most relevant to its detection is the next challenge. From my perspective, if a clinical trial of a treatment shows that it is effective in objective indicators but not as successful in more subjective aspects of quality of life, response-shift effects may be playing a role in this paradox.
Nonetheless, savoring this success and the success of the subfield of response-shift research is something we should do. And thanking our beloved societal “home” – ISOQOL – is crucial. For ISOQOL gave us response-shift methodologists an environment in which to grow our community, and from which many seminal response-shift collaborations emerged. So, thank you, ISOQOL!
Corresponding author
Carolyn Schwartz, Sc.D., DeltaQuest Foundation, Inc.
31 Mitchell Road
Concord, MA 01742
carolyn.schwartz@deltaquest.org
Funding
No funding was used for this editorial.
Notes
Role of the funder: Not applicable.
Author Disclosures: CES has no disclosures.
Author contributions: CES: writing – original draft, writing – review & editing.
Data Availability: No new data were generated or analyzed for this editorial.
This newsletter editorial represents the views of the author and does not necessarily reflect the views of ISOQOL.
How to Submit a Newsletter Editorial
Do you have something to share about health related quality of life and patient-centered outcomes? We want to hear from you!
Learn More
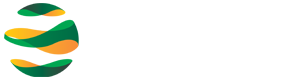
The International Society for Quality of Life Research (ISOQOL) is a global community of researchers, clinicians, health care professionals, industry professionals, consultants, and patient research partners advancing health related quality of life research (HRQL).
Together, we are creating a future in which patient perspective is integral to health research, care and policy.